Rice D2K Lab students working with Texas Children’s Hospital created machine-learning algorithms to increase detection time before cardiac arrest
Cardiac arrest events are often preceded by subtle, almost undetectable signs. What if doctors can detect early signals faster and more accurately with deep-learning algorithms?
Team Cardiac Signals, six senior electrical and computer engineering majors at Rice University, developed a metric to give an early warning for cardiac events by analyzing electrocardiogram (ECG) signals. “This metric can make life-saving treatments proactive instead of reactive,” said Frank Yang, a physics major.
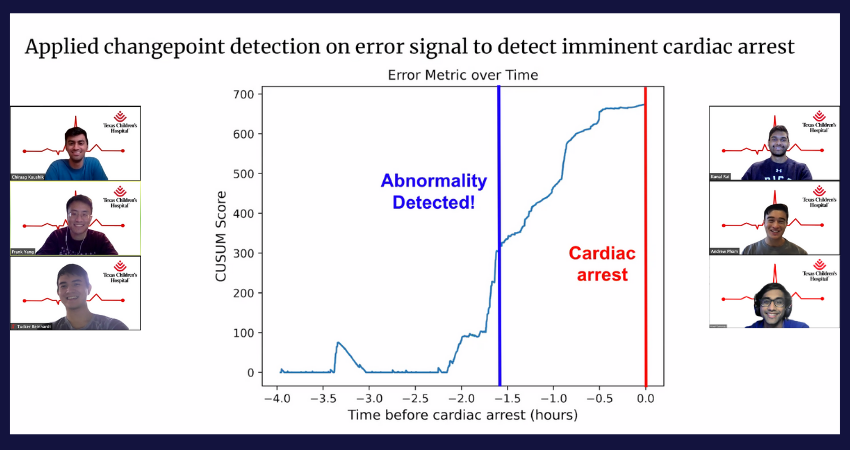
(Team Cardiac Signals won first place in the Fall 2020 D2K Virtual Showcase. From top left: Chiraag Kaushik, Frank Yang, and Tucker Reinhart. From top right: Kunal Rai, Andrew Pham, and Aneel Damaraju.)
Sponsored by Texas Children’s Hospital, the physiological data were collected from 44 pediatric cardiac patients. Each was diagnosed with hypoplastic left heart syndrome (HLHS), a condition in which babies are born with only half of their hearts developed. Patients’ survival depended on cardiologists’ early detection of abnormalities.
The student team used auto-encoders, non-linear deep-learning algorithms to monitor the ECG heartbeats before cardiac arrest. “We learned the patterns of healthy heartbeats and compared other heartbeats to assess how well they followed these patterns,” Damaraju said. “Then, we ultimately detected when heartbeats began to deviate from healthy behavior which indicated cardiac electrical stability.”
The detection model the team developed predicted cardiac arrest in 73 percent of the tested patients, with a detection time of 1.3 hours prior to the event. “The time for a doctor to tell irregular ECG signals is usually 20 minutes or so before the cardiac arrest, which doesn’t give them a lot of reaction time to do anything proactively,” said Kaushik. “So 1.3 hours is a significant improvement that would give them more time to save lives.”
The team is looking to continue working on this project and testing their model with more patient data in the next semester. Yang said, “Our team complimented each other well. We have a mix of backgrounds, where some people are great resources for the programming portion and others can focus on modeling or literature review.”
Besides a diverse skill set, most team members are double majors. Damaraju and Yang also study physics; Reinhart and Pham, computer science majors. “The cool thing about the Capstone program is that we actually help produce something useful, especially something like predicting cardiac signals, that I never would have thought I would be doing as a computer science and electrical engineering major,” Reinhart said.
“This project has been an amazing culmination of my studies at Rice over the past four years,” Pham added. “Working on real-world challenges was a great way to prepare for my transition from school to my career.”
Watch Team Cardiac Signals’ video to learn more about their work:
https://www.youtube.com/watch?v=oy-TLHmD-Ac
Interested in sponsoring a D2K Capstone project?
Visit the website to learn more: Rice Data Science Capstone Program.