Do you know that every 40 seconds someone in the US has a stroke? If a patient has a recurrent or additional stroke, they are 17 times more likely to die - which is why early detection and mitigation of recurrent stroke is critical to reducing the overall stroke fatality rate.
D2K student team "Stroke Risk" worked on a data science capstone project sponsored by UTHealth to predict recurrent strokes using machine learning. Team members were six senior electrical and computer engineering (ECE) students: Artun Bayer, Josue Casco-Rodriguez, Nick Glaze, Samantha Fuentes, Justin Cheung, and Michael Sprintson
Students leveraged UTHealth’s comprehensive database of nearly 5,000 stroke patients. This dataset contained a variety of data: electronic health records, brain scan images, and texts of clinical notes.
In the first semester of their year-long project, the team converted the EHR data into a graph, started extracting features from the image and text data, and trained some graph learning models that can predict recurrent stroke. Their project won Honorable Mention in the Fall 2021 D2K Showcase.
Updated on April 25 2022:
"This semester we added two more modalities," said Michael Sprintson '22 ECE. "Not only did we add the notes modality, but we also had over 3/4 of a million individual uncrossed text documents. We also added image modality, which is a huge amount of CT and MRI scans. Together, these were processed into the same graph framework to help produce a final prediction." UTHealth can use this framework to identify at-risk patients, give them the preventative care they need, and ultimately save their lives.
Their project won first place in the Spring 2022 Showcase.
Watch their 1-min pitch video.
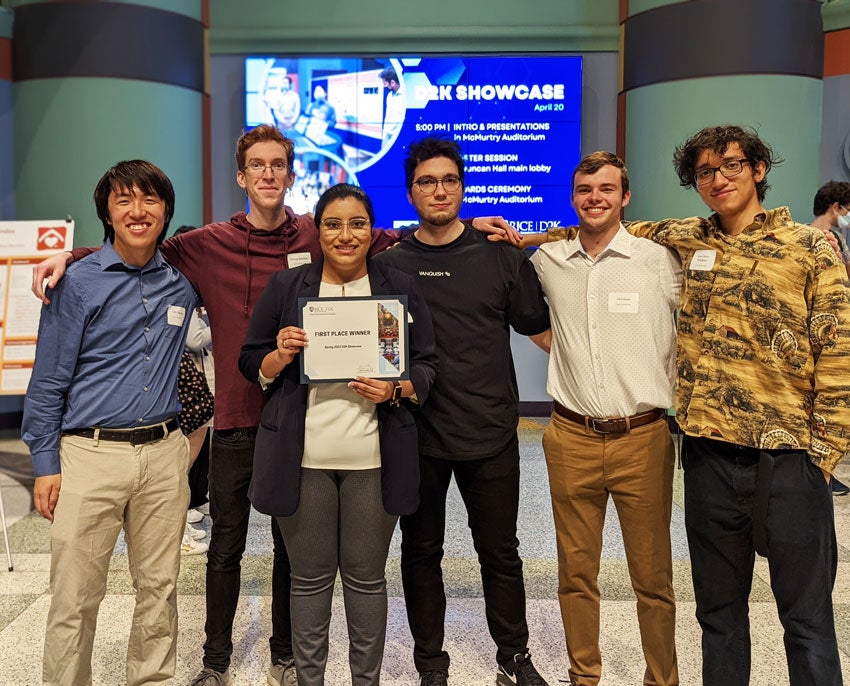