Do you know that unexpected shutdowns in the natural gas compression industry cost millions of dollars every year? Texas is an energy-powered state, where having a reliable power grid impacts everyone’s bottom line.
Five Rice engineering students, enrolled in the data science projects capstone offered by the D2K Lab and sponsored by D2K Lab Affiliate member CSI Compressco, worked with compressor data sets and used predictive modeling to prevent costly shutdowns. Team CS I Predict competed at the end of semester Spring 2021 D2K Showcase and won first place.
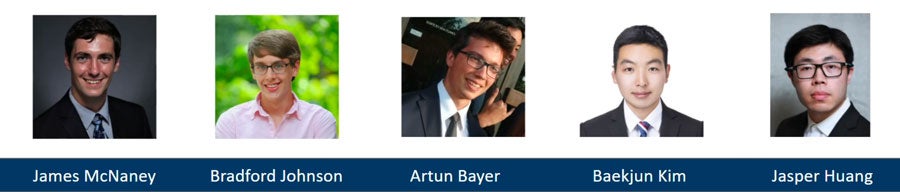
The team members are master’s students James McNaney, in electrical and computer engineering (ECE), Baekjun Kim in computer science (CS), and undergraduates Bradford Johnson in computational and applied mathematics (CAAM), Artun Bayer in electrical and computer engineering (ECE) and Jasper Huang in statistics (STAT).
The natural gas streamline process has existed for many years, but with the help of machine learning, Team CS I Predict aims to make the process even more efficient.
“Every shutdown we can predict ahead of time helps not only CSI Compressco but the population as a whole,” said Brad Johnson, a senior graduating in the fall.
Challenges and Solutions
A large amount of processing is required to make the industrial data usable. Some of the data processing challenges of this project include changing the formats, filtering the data and processing those values.
“Splitting the roles and doing our best on each part allowed us to finish the project on time. It was an exciting experience, and I was able to feel the synergy of the team play,” said Baekjun Kim, a master in CS student graduating in the fall.
Just like working on a complex real-world project for any industry, being able to adjust and collaborate quickly makes a big difference to the final results.
Recent alumnus Jasper Huang (‘21 STAT) shared, “Something that's really helpful is we looked at very similar projects and what they did successfully, which helped us narrow down our methodology into what we wanted to focus on.”
Master in ECE student James McNaney shared, “This mindset of having to frame and justify our engineering decisions in an understandable, persuasive manner also helped shape the direction of our project. I thought the Capstone experience was an invaluable, first-hand example of keeping the "big picture" in mind when tackling a real-world problem.”
Interdisciplinary Collaboration
The D2K Capstone program brings together interdisciplinary teams of students (undergraduate and graduate students) to work on data science projects sponsored by industry, community partners and researchers. For Team CS I Predict, all of the members were from different majors in Rice engineering. “We didn't have strictly defined roles because it was more like what we felt comfortable contributing most and doing the best of my ability to help,” said Jasper Huang. “I have experience with coding, so I helped with some data explorations, like the ROC curve part of our presentation.”
“This is my first time working on a team for the entire semester,” Baekjun Kim said. “Especially for professional master students who want to further their career, you will learn ideas and perspectives from all academic backgrounds in the Capstone program.”
Mentorship from D2K faculty plays an important role throughout the semester. “Having the mentorship of Drs. Arko Barman and Genevera Allen helped us quite a bit in coming to what result we had. Their guidance pushed us in the right direction,” Johnson said.
Results and Impact
The team wrangled reams of minute-level sensor readings and telemetry data on compressor units and used machine learning to find patterns indicative of shutdowns. The team built an autoencoder neural network model to identify anomalies in the sensor readings that could predict a shutdown 115 minutes in advance with a detection rate of 60 percent.
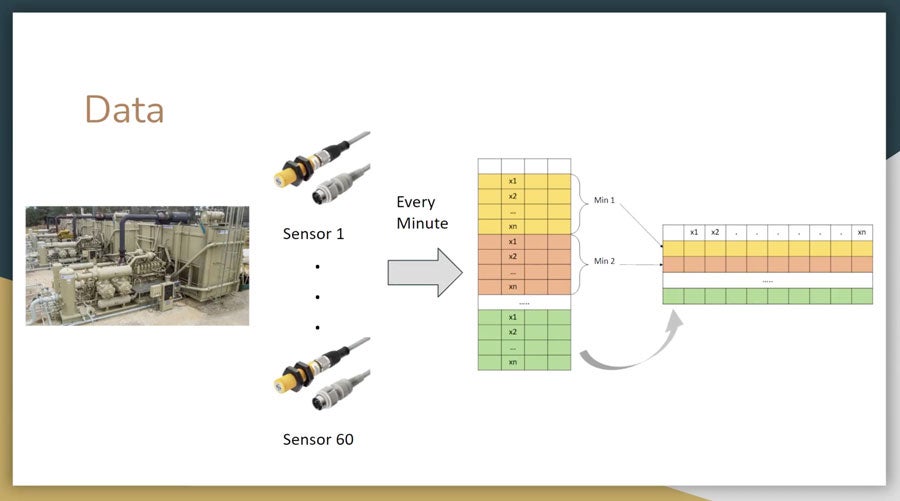
They separated the data into two categories to train their model and normal operation data. They took four-hour windows leading up to each shutdown, the shutdown data, and used the remaining data as normal which were split into one-hour windows.
It is important to note that they were able to pinpoint which types of shutdowns were being correctly detected across all machines. With additional reporting of the returning specific features, technicians will be able to determine the class and severity of a shutdown.
This project not only helps CSI Compressco protect their machines and keep their customers happy, but also helps the general population by potentially raising the reliability of the entire energy grid.
“We are very proud of this team for working on an extremely challenging open problem with large, messy data sets from the energy industry and deriving valuable solutions,” D2K Founder and Faculty Director, Genevera Allen adds.
Watch their video highlight to learn more >>
To view all the Spring 2021 Capstone projects, visit the D2K Showcase page.
Interested in sponsoring a Capstone project for Fall 2021? Contact d2k@rice.edu for more information.