Advanced data science students at Rice University helped a global medical technology company determine optimal battery replacement timelines for a device used to treat epilepsy.
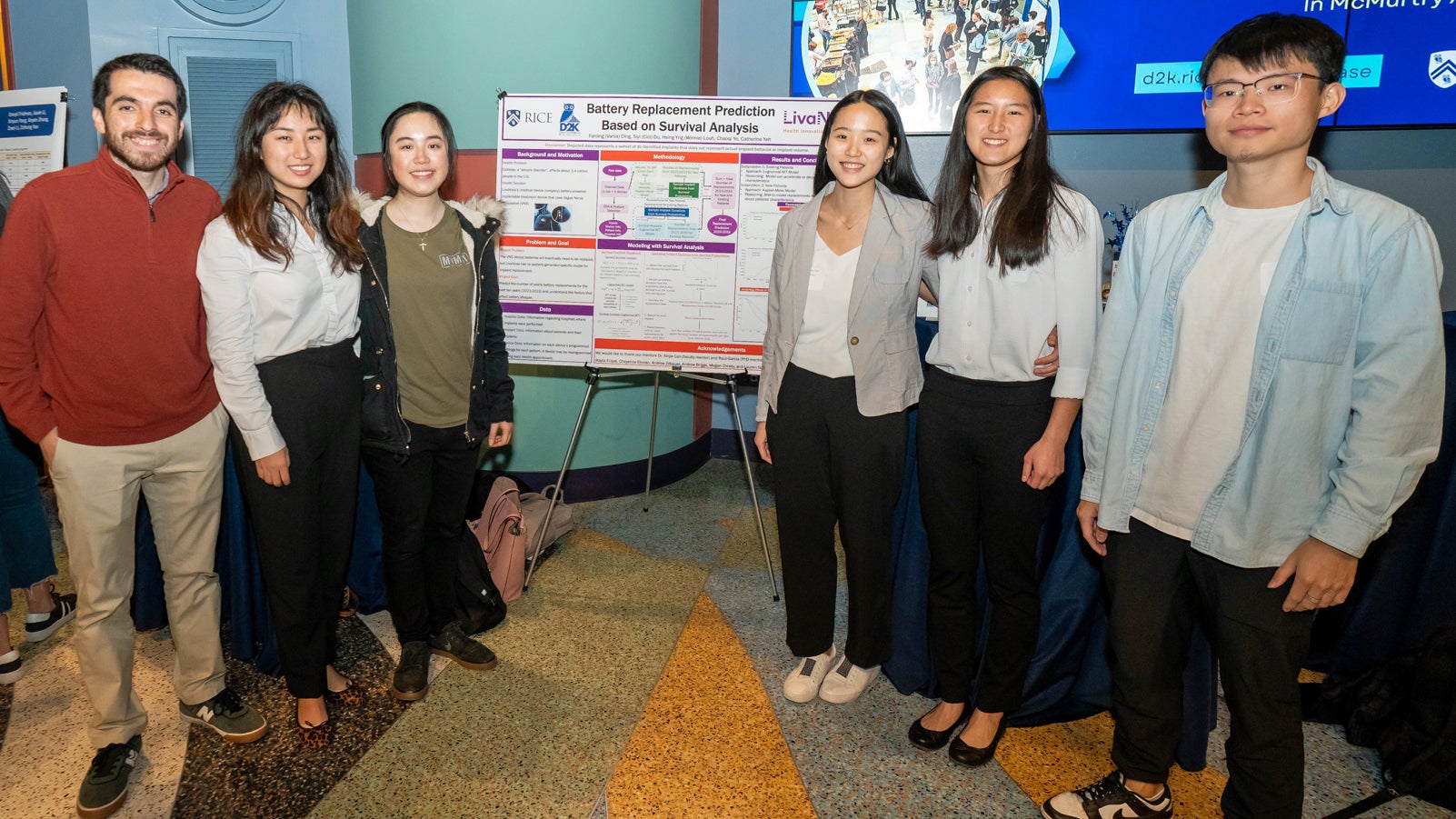
Drug-resistant epilepsy impacts almost a third of the 3.4 million people in the U.S. experiencing the brain disorder. With these epilepsy patients in mind, LivaNova developed a battery-operated implant that stimulates the vagus nerve. There is not an accurate battery depletion tracker on the device, so students were charged with building a statistical model to determine how much battery remains for both active and potential future devices.
Xinjie Lan, the D2K faculty mentor for Team LivaNova, said, “We aim to apply data science to help people with epilepsy. Students collaborated with LivaNova to build a statistical model to forecast battery replacements for epilepsy patients in the next 10 years. They worked incredibly hard and produced a thoughtful final presentation.”
The Rice students on Team LivaNova earned the judges’ highest score in the fall 2022 Data to Knowledge (D2K) Showcase. From the students’ model, LivaNova can better inform physicians and patients when their battery is nearing depletion.
“Team LivaNova was tasked with a challenging problem and given multiple messy data sources,” said Kayla Frisoli, LivaNova data scientist and senior manager for competitive intelligence and data analytics. “They needed to truly understand the business context in order to properly handle the data and modeling implications. Their problem involved developing and aggregating different models for both new and existing patients to create a single forecast. These models required different approaches as the factors available in each setting differed."
“While many students can build statistical machine learning models, Team LivaNova evolved into true data scientists throughout this project developing business acumen, properly cleaning the data, building a model and interpreting it within context, and communicating their results effectively to a wide range of audiences. We were thrilled to see this development of the students throughout the semester and wish them the best of luck in their next endeavors.”
Bill.com’s duplicate vendor entries solution
Competition for the judges’ top score was strong and the Bill.com student team helped resolve a problem with duplicate vendors to win the first runner up spot.
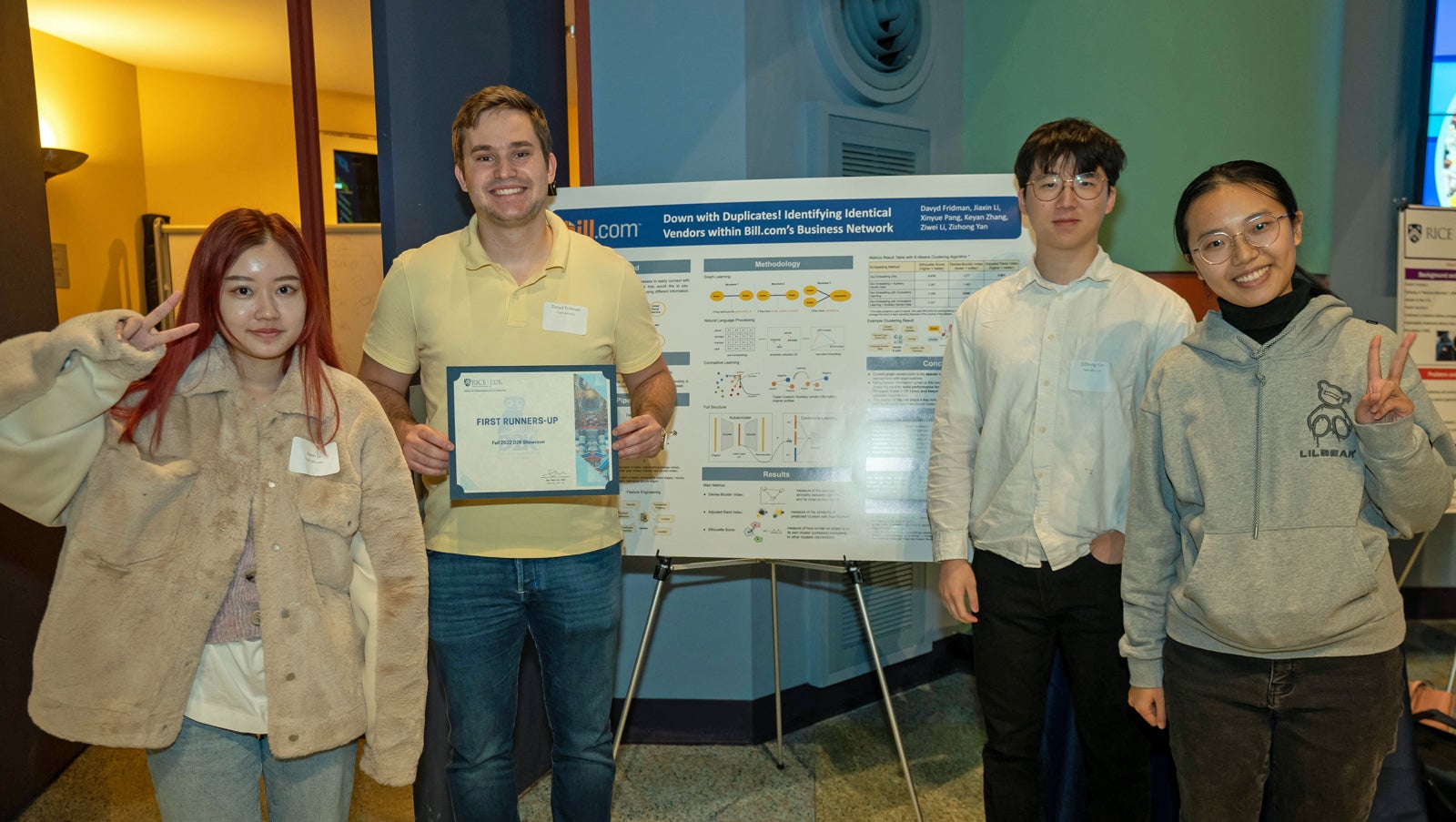
One of the services Bill.com provides their clients is a simplified vendor payment process. However, the free text entry each client uses to set up their own vendors may result in duplicates in the system. To speed up the vendor search function, Bill.com submitted their challenge to Rice students. Using a machine learning algorithm, natural language processing, auto-encoders, and other tools, Team Bill.com provided a solution to identify and eliminate duplicate vendors.
Michael Price, a machine learning engineer at Bill.com, said, “My biggest surprise was how the students utilized the completely different data sources we provided. The students were able to combine graph, text and tabular data to create an effective deduplication algorithm. Standard data science courses usually do not teach how to incorporate different data types, so we are extremely happy that the students thought outside the box and explored many unconventional methods.”
Bill.com manager of Network and Payments ML Ricardo Fernandez was also impressed with the Rice team’s ability to combine structured and unstructured data into a single model. He said, “The students did a great job in thinking outside the box. They found initiative ways to combine the data and make a unique solution."
“I was also impressed by how the students cycled through many ideas, many new to them. As a group, they delegated tasks efficiently and quickly were able to assess the virtues of the methods in question.”
Rice Electrical Engineering assistant professor Arko Barman serves as a D2K faculty advisor to several of the capstone teams each semester. He said, “The Bill.com team were tasked with a very challenging problem and they showed great creativity to leverage advanced state-of-the-art AI concepts to design their own novel solution."
Houston Fire Department data analysis helps avoid future traffic incidents
Barman also served as the faculty advisor to the team partnered with the Houston Fire Department, a sponsor who has participated in every showcase since 2019. Their student team earned the second runner up award with their work on identifying likely causes of traffic collisions impacting HFD vehicles.
He said, “We have a responsibility to use data science and machine learning for social good. The students have done exactly that to help HFD identify, analyze, and possibly prevent incidents, to operate their fleet more safely.”
The students on the team were eager to use their data science skills to help local firefighters identify the conditions, locations, time of day, and other contributing factors that can lead to delays in response time caused by traffic collisions.
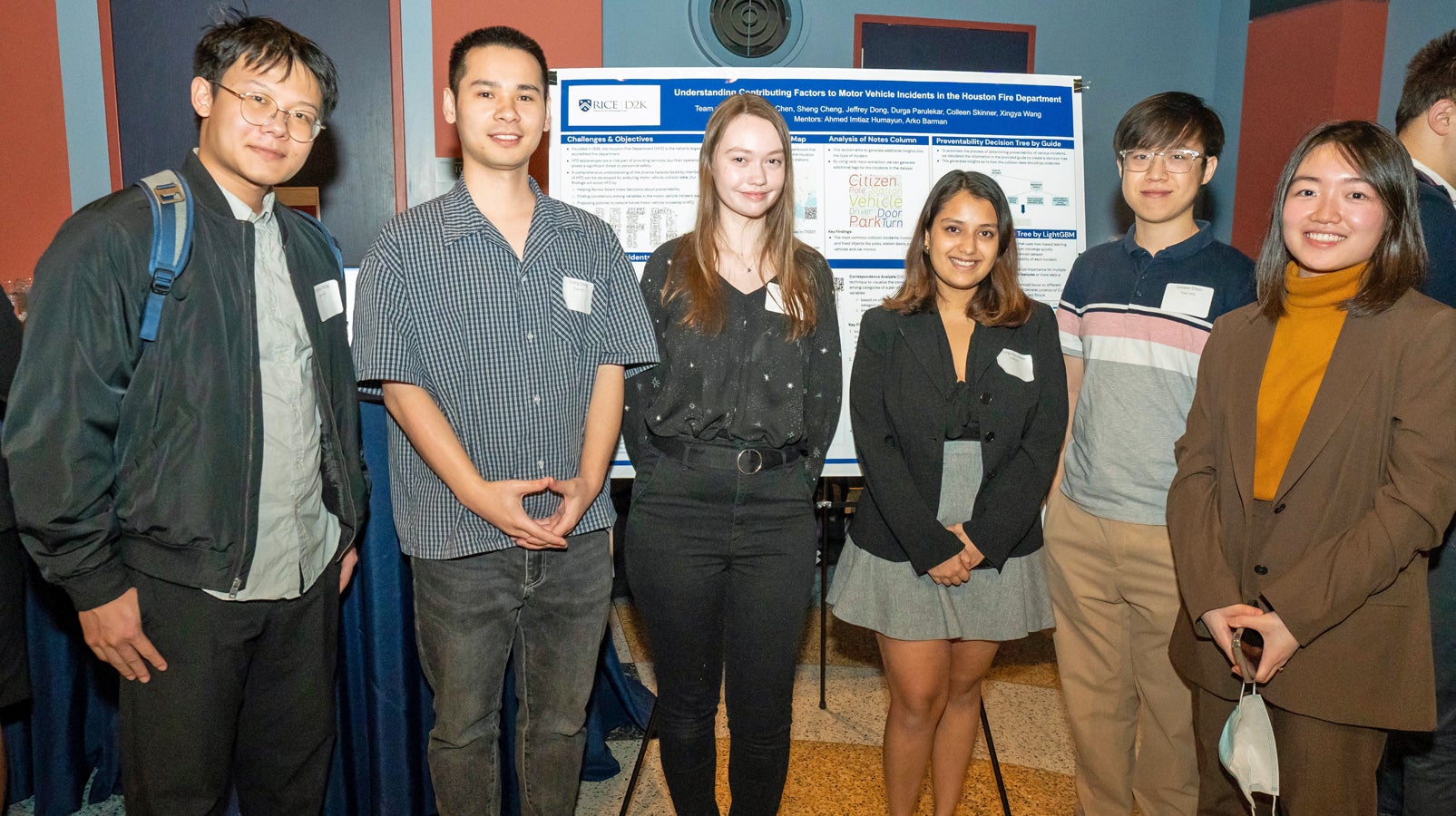
Leonard Chan, HFD accreditation manager, said, “For years, the Rice D2K program has provided technical expertise to support the Houston Fire Department. This semester was no exception as the team helped visually illustrate the conditions surrounding collisions involving department vehicles, including our fire trucks and ambulances."
“The Rice D2K program has continued to demonstrate for us the potential our wealth of data has for conversion into meaningful actions that can improve our operational effectiveness and, in turn, improve the public safety of Houston. In fact, since our partnership with the Rice D2K program, HFD has emerged as one of the pioneers in the use of fire service data. Fire departments across North America have been interested in the data Rice D2K is analyzing on our behalf and want to know what is next.”
Congratulations to all the winning teams!
Interested in working with Rice student and faculty teams to solve real-world data science challenges?
Email d2k@rice.edu for more information about the D2K Capstone projects.